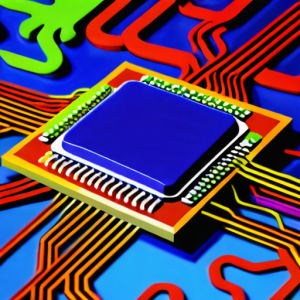
The U.S. FDA, Health Canada, and the UK’s MHRA have unveiled 10 guiding principles for Good Machine Learning Practice (GMLP) in developing AI/ML medical devices. These principles aim to ensure safety, efficacy, and quality in healthcare innovation. Key focuses include leveraging multi-disciplinary expertise, implementing good software and security practices, ensuring representative clinical study participants and data sets, maintaining independence between training and test data sets, and emphasizing the performance of the human-AI team. These guidelines also highlight the importance of clear user information, robust testing, and ongoing monitoring of deployed models to manage re-training risks and maintain performance.
Read the full GMLP draft on the FDA website.
1-Minute Summary
Here are the ten principles of GMLP.
- Leverage Multi-Disciplinary Expertise for ML-Enabled Medical Devices: Ensuring safe, effective models through comprehensive integration and understanding clinical workflows, benefits, and risks.
- Implement Good Software Engineering and Security Practices: Focus on data integrity, quality, and cybersecurity to ensure robust and safe model design and implementation.
- Ensure Clinical Study Participants Represent Intended Population: Collect diverse data reflecting intended users to manage bias and generalize performance, improving model reliability.
- Maintain Independence Between Training and Test Data Sets: Ensure datasets are independent to avoid bias, considering all sources of dependence for accurate model evaluation.
- Utilize Best Methods for Reference Datasets: Adopt clinically relevant and characterized data, using accepted reference standards for robust and generalizable model testing.
- Tailor Model Design to Data and Intended Use: Design models suited to data and intended use, focusing on mitigating risks and achieving clinically meaningful goals.
- Emphasize Performance of Human-AI Team: Address human factors and model interpretability, prioritizing the combined performance of humans and AI in clinical settings.
- Test Device Performance Under Clinically Relevant Conditions: Develop and execute test plans reflecting real-world use, considering patient populations and clinical environments for accurate performance assessment.
- Provide Clear, Essential Information to Users: Offer accessible, relevant information to users, detailing model use, performance, training data characteristics, and integration into clinical workflows.
- Monitor Deployed Models for Performance and Manage Re-training Risks: Ensure deployed models are monitored for safety and performance, with controls for re-training to manage risks of bias and degradation.
Applying GMLP Principles to Radiology
The advent of Good Machine Learning Practice (GMLP) guidelines is a guide for the future of radiology, toward integrating artificial intelligence and machine learning (AI/ML) into clinical imaging practices. Radiology stands at the forefront of specialties poised to benefit from AI’s diagnostic precision, efficiency improvements, and the capacity to uncover insights buried within imaging data. These new guiding principles offer a framework for ensuring that AI/ML technologies are developed and implemented in safe, effective, and beneficial ways for patient care.
Without taking too much of your time, here are three applications worth highlighting for radiology.
Multidisciplinary Approach to Machine Learning
For radiologists, the GMLP guidelines underscore the importance of a multidisciplinary approach to AI development, where clinical insights from radiology are crucial for shaping AI tools that are both clinically relevant and aligned with patient needs. The emphasis on data representativeness and managing bias is particularly pertinent, given radiology’s reliance on diverse datasets to train algorithms capable of recognizing a wide range of pathologies across different populations.
The Human-AI Team as Good Practice
The guidelines also highlight the critical role of the human-AI team, reinforcing the idea that AI should augment rather than replace the radiologist’s expertise. This synergy between human and machine can enhance diagnostic accuracy, reduce errors, and allow radiologists to focus on more complex interpretative and decision-making tasks. I have also recently written about how a radiologist-AI team could operate as a Centaur and/or as a Cyborg.
Ongoing Monitoring for AI/ML Devices
Moreover, the principles of ongoing performance monitoring and the adaptability of deployed models speak directly to the dynamic nature of radiology. As imaging technologies and clinical practices evolve, so too must the AI/ML tools we rely on, ensuring they remain effective and responsive to new challenges and opportunities.
Incorporating these principles into radiology’s embrace of AI/ML promises not just to advance the field technologically but also to ensure that these advancements are achieved responsibly, with patient welfare at their core. Radiology professionals should engage with these guidelines actively, using them as a blueprint for integrating AI into their work in ethical, effective, and transformative ways for patient care.