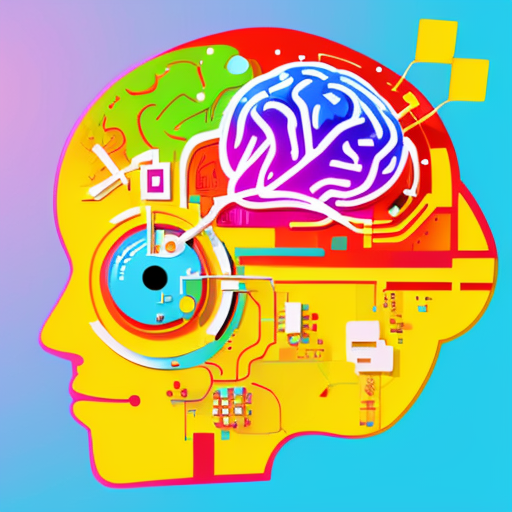
In the rapidly evolving field of radiology, artificial intelligence (AI) is not just a tool but a collaborator, reshaping the dynamics of diagnosis and patient care. On the first order, the answer seemed clear: knowledge workers using AI outperforms those that don’t.
But the literature offers little detail on what happens after you embrace AI. Just with every tool ever existed, it really matters how you use it. As it turns out, it also matters how AI becomes part of your work.
To better understand this partnership, a group of Harvard Business School scholars published a study on business consultants who have, and who have not, opted to adopt GPT-4 in their daily work in spring 2023. There are several interesting conclusions – one of them delve into the analogy of centaurs versus cyborgs, concepts borrowed from mythology and science fiction that provide a vivid framework for the interaction between human intelligence and AI in radiology.
Continue reading